Stealthy Linux rootkit found in the wild after going undetected for 2 years
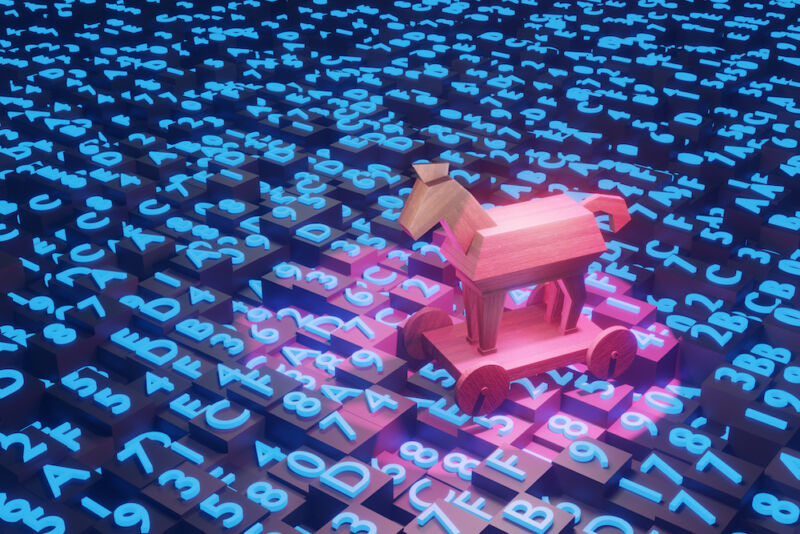
Stealthy and multifunctional Linux malware that has been infecting telecommunications companies went largely unnoticed for two years until being documented for the first time by researchers on Thursday.
Researchers from security firm Group-IB have named the remote access trojan “Krasue,” after a nocturnal spirit depicted in Southeast Asian folklore “floating in mid-air, with no torso, just her intestines hanging from below her chin.” The researchers chose the name because evidence to date shows it almost exclusively targets victims in Thailand and “poses a severe risk to critical systems and sensitive data given that it is able to grant attackers remote access to the targeted network.
According to the researchers:
- Krasue is a Linux Remote Access Trojan that has been active since 20 and predominantly targets organizations in Thailand.
- Group-IB can confirm that telecommunications companies were targeted by Krasue.
- The malware contains several embedded rootkits to support different Linux kernel versions.
- Krasue’s rootkit is drawn from public sources (3 open-source Linux Kernel Module rootkits), as is the case with many Linux rootkits.
- The rootkit can hook the `kill()` syscall, network-related functions, and file listing operations in order to hide its activities and evade detection.
- Notably, Krasue uses RTSP (Real-Time Streaming Protocol) messages to serve as a disguised “alive ping,” a tactic rarely seen in the wild.
- This Linux malware, Group-IB researchers presume, is deployed during the later stages of an attack chain in order to maintain access to a victim host.
- Krasue is likely to either be deployed as part of a botnet or sold by initial access brokers to other cybercriminals.
- Group-IB researchers believe that Krasue was created by the same author as the XorDdos Linux Trojan, documented by Microsoft in a March 2022 blog post, or someone who had access to the latter’s source code.
During the initialization phase, the rootkit conceals its own presence. It then proceeds to hook the `kill()` syscall, network-related functions, and file listing operations, thereby obscuring its activities and evading detection.
The researchers have so far been unable to determine precisely how Krasue gets installed. Possible infection vectors include through vulnerability exploitation, credential-stealing or -guessing attacks, or by unwittingly being installed as trojan stashed in an installation file or update masquerading as legitimate software.
The three open source rootkit packages incorporated into Krasue are:
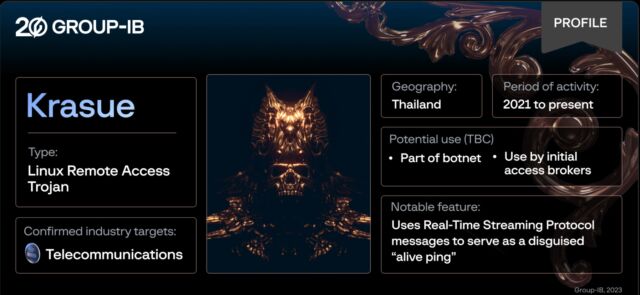
Enlarge / An image showing salient research points of Krasue.
Group-IB
Rootkits are a type of malware that hides directories, files, processes, and other evidence of its presence to the operating system it’s installed on. By hooking legitimate Linux processes, the malware is able to suspend them at select points and interject functions that conceal its presence. Specifically, it hides files and directories beginning with the names “auwd” and “vmware_helper” from directory listings and hides ports 52695 and 52699, where communications to attacker-controlled servers occur. Intercepting the kill() syscall also allows the trojan to survive Linux commands attempting to abort the program and shut it down.
Stealthy Linux rootkit found in the wild after going undetected for 2 years Read More »