GM uses AI tool to determine which truck stops should get EV chargers
help me choose —
Forget LLM chatbots; this seems like an actually useful implementation of AI.
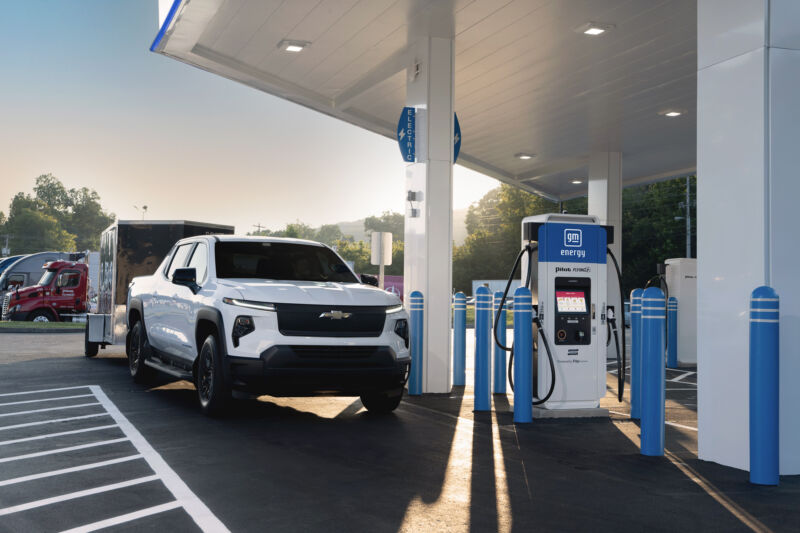
Enlarge / A 2024 Chevrolet Silverado EV WT at a pull-through charging stall located at a flagship Pilot and Flying J travel center, as part of the new coast-to-coast fast charging network.
General Motors
It’s understandable if you’re starting to experience AI fatigue; it feels like every week, there’s another announcement of some company boasting about how an LLM chatbot will revolutionize everything—usually followed in short succession by news reports of how terribly wrong it’s all gone. But it turns out that not every use of AI by an automaker is a public relations disaster. As it happens, General Motors has been using machine learning to help guide business decisions regarding where to install new DC fast chargers for electric vehicles.
GM’s transformation into an EV-heavy company has not gone entirely smoothly thus far, but in 2022, it revealed that, together with the Pilot company, it was planning to deploy a network of 2,000 DC fast chargers at Flying J and Pilot travel centers around the US. But how to decide which locations?
“I think that the overarching theme is we’re really looking for opportunities to simplify the lives of our customers, our employees, our dealers, and our suppliers,” explained Jon Francis, GM’s chief data and analytics officer. “And we see the positive effects of AI at scale, whether that’s in the manufacturing part of the business, engineering, supply chain, customer experience—it really runs through threads through all of those.
“Obviously, the place where it shows up most directly is certainly in autonomous, and that’s an important use case for us, but actually [on a] day-to-day basis, AI is improving a lot of systems and workflows within the organization,” he told Ars.
“There’s a lot of companies—and not to name names, but there’s some chasing of shiny objects, and I think there are a lot of cool, sexy things that you can do with AI, but for GM, we’re really looking for solutions that are going to drive the business in a meaningful way,” Francis said.
GM wants to build out chargers at about 200 Flying J and Pilot travel centers by the end of 2024, but narrowing down exactly which locations to focus on was the big question. After all, there are more than 750 spread out across 44 US states and six Canadian provinces.
Obviously, traffic is a big concern—each DC fast charger costs anywhere from $100,000 to $300,000 dollars, and that’s not counting any costs associated with beefing up the electrical infrastructure to power them, nor the various permitting processes that tend to delay everything. Sticking a bank of chargers at a travel center that’s rarely visited isn’t the best use of resources, but neither is deploying them in an area that’s already replete with other fast chargers.
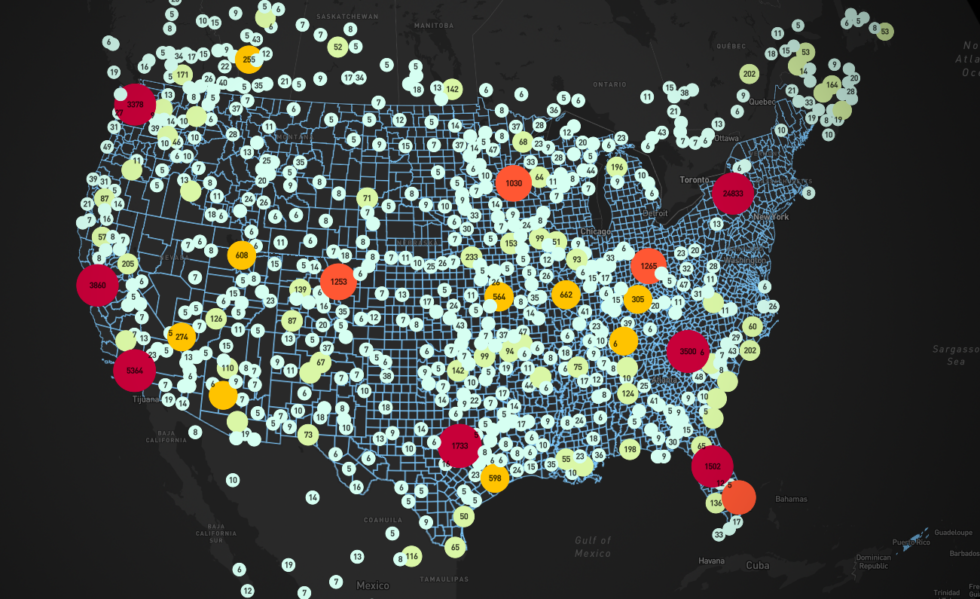
Enlarge / Much of the data GM showed me was confidential, but this screenshot should give you an idea of how the various datasets combine.
General Motors
Which is where the ML came in. GM’s data scientists built tools that aggregate different GIS datasets together. For example, it has a geographic database of already deployed DC chargers around the country—the US Department of Energy maintains such a resource—overlayed with traffic data and then the locations of the travel centers. The result is a map with potential locations, which GM’s team then uses to narrow down the exact sites it wants to choose.
It’s true that if you had access to all those datasets, you could probably do all that manually. But we’re talking datasets with, in some cases, billions of data points. A few years ago, GM’s analysts could have done that at a city level without spending years on the project, but doing it on a nationwide scale is the kind of task that requires the amount of cloud platforms and distributed clusters that are really now only becoming commonplace.
As a result, GM was able to deploy the first 25 sites last year, with 100 charging stalls across the 25. By the end of this year, it told Ars it should have around 200 locations operational.
That certainly seems more useful to me than just another chatbot.
GM uses AI tool to determine which truck stops should get EV chargers Read More »