Lightening the load: AI helps exoskeleton work with different strides
One model to rule them all —
A model trained in a virtual environment does remarkably well in the real world.
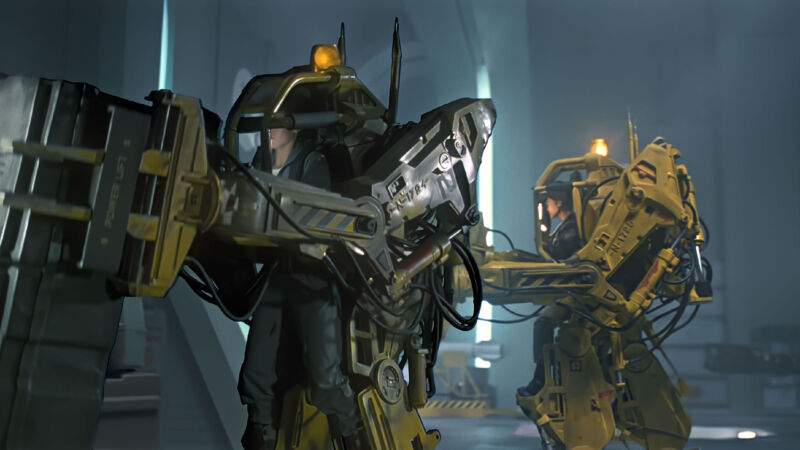
Enlarge / Right now, the software doesn’t do arms, so don’t go taking on any aliens with it.
20th Century Fox
Exoskeletons today look like something straight out of sci-fi. But the reality is they are nowhere near as robust as their fictional counterparts. They’re quite wobbly, and it takes long hours of handcrafting software policies, which regulate how they work—a process that has to be repeated for each individual user.
To bring the technology a bit closer to Avatar’s Skel Suits or Warhammer 40k power armor, a team at North Carolina University’s Lab of Biomechatronics and Intelligent Robotics used AI to build the first one-size-fits-all exoskeleton that supports walking, running, and stair-climbing. Critically, its software adapts itself to new users with no need for any user-specific adjustments. “You just wear it and it works,” says Hao Su, an associate professor and co-author of the study.
Tailor-made robots
An exoskeleton is a robot you wear to aid your movements—it makes walking, running, and other activities less taxing, the same way an e-bike adds extra watts on top of those you generate yourself, making pedaling easier. “The problem is, exoskeletons have a hard time understanding human intentions, whether you want to run or walk or climb stairs. It’s solved with locomotion recognition: systems that recognize human locomotion intentions,” says Su.
Building those locomotion recognition systems currently relies on elaborate policies that define what actuators in an exoskeleton need to do in each possible scenario. “Let’s take walking. The current state of the art is we put the exoskeleton on you and you walk on a treadmill for an hour. Based on that, we try to adjust its operation to your individual set of movements,” Su explains.
Building handcrafted control policies and doing long human trials for each user makes exoskeletons super expensive, with prices reaching $200,000 or more. So, Su’s team used AI to automatically generate control policies and eliminate human training. “I think within two or three years, exoskeletons priced between $2,000 and $5,000 will be absolutely doable,” Su claims.
His team hopes these savings will come from developing the exoskeleton control policy using a digital model, rather than living, breathing humans.
Digitizing robo-aided humans
Su’s team started by building digital models of a human musculoskeletal system and an exoskeleton robot. Then they used multiple neural networks that operated each component. One was running the digitized model of a human skeleton, moved by simplified muscles. The second neural network was running the exoskeleton model. Finally, the third neural net was responsible for imitating motion—basically predicting how a human model would move wearing the exoskeleton and how the two would interact with each other. “We trained all three neural networks simultaneously to minimize muscle activity,” says Su.
One problem the team faced is that exoskeleton studies typically use a performance metric based on metabolic rate reduction. “Humans, though, are incredibly complex, and it is very hard to build a model with enough fidelity to accurately simulate metabolism,” Su explains. Luckily, according to the team, reducing muscle activations is rather tightly correlated with metabolic rate reduction, so it kept the digital model’s complexity within reasonable limits. The training of the entire human-exoskeleton system with all three neural networks took roughly eight hours on a single RTX 3090 GPU. And the results were record-breaking.
Bridging the sim-to-real gap
After developing the controllers for the digital exoskeleton model, which were developed by the neural networks in simulation, Su’s team simply copy-pasted the control policy to a real controller running a real exoskeleton. Then, they tested how an exoskeleton trained this way would work with 20 different participants. The averaged metabolic rate reduction in walking was over 24 percent, over 13 percent in running, and 15.4 percent in stair climbing—all record numbers, meaning their exoskeleton beat every other exoskeleton ever made in each category.
This was achieved without needing any tweaks to fit it to individual gaits. But the neural networks’ magic didn’t end there.
“The problem with traditional, handcrafted policies was that it was just telling it ‘if walking is detected do one thing; if walking faster is detected do another thing.’ These were [a mix of] finite state machines and switch controllers. We introduced end-to-end continuous control,” says Su. What this continuous control meant was that the exoskeleton could follow the human body as it made smooth transitions between different activities—from walking to running, from running to climbing stairs, etc. There was no abrupt mode switching.
“In terms of software, I think everyone will be using this neural network-based approach soon,” Su claims. To improve the exoskeletons in the future, his team wants to make them quieter, lighter, and more comfortable.
But the plan is also to make them work for people who need them the most. “The limitation now is that we tested these exoskeletons with able-bodied participants, not people with gait impairments. So, what we want to do is something they did in another exoskeleton study at Stanford University. We would take a one-minute video of you walking, and based on that, we would build a model to individualize our general model. This should work well for people with impairments like knee arthritis,” Su claims.
Nature, 2024. DOI: 10.1038/s41586-024-07382-4
Lightening the load: AI helps exoskeleton work with different strides Read More »