IV infusion enables editing of the cystic fibrosis gene in lung stem cells
Right gene in the right place —
Approach relies on lipid capsules like those in the mRNA vaccines.
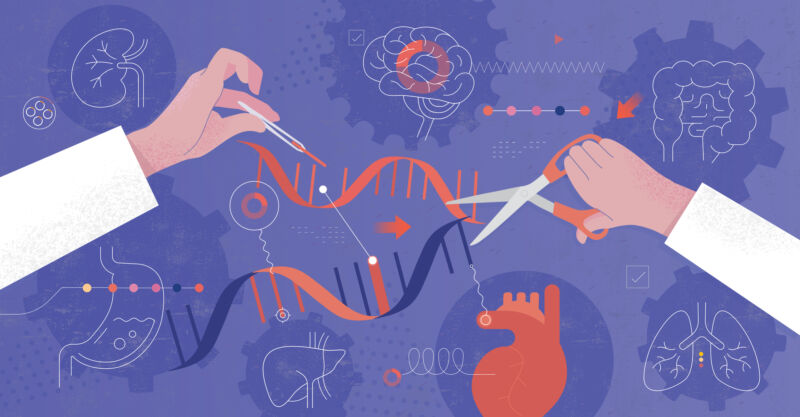
The development of gene editing tools, which enable the specific targeting and correction of mutations, hold the promise of allowing us to correct those mutations that cause genetic diseases. However, the technology has been around for a while now—two researchers were critical to its development in 2020—and there have been only a few cases where gene editing has been used to target diseases.
One of the reasons for that is the challenge of targeting specific cells in a living organism. Many genetic diseases affect only a specific cell type, such as red blood cells in sickle-cell anemia, or specific tissue. Ideally, to limit potential side effects, we’d like to ensure that enough of the editing takes place in the affected tissue to have an impact, while minimizing editing elsewhere to limit side effects. But our ability to do so has been limited. Plus, a lot of the cells affected by genetic diseases are mature and have stopped dividing. So, we either need to repeat the gene editing treatments indefinitely or find a way to target the stem cell population that produces the mature cells.
On Thursday, a US-based research team said that they’ve done gene editing experiments that targeted a high-profile genetic disease: cystic fibrosis. Their technique largely targets the tissue most affected by the disease (the lung), and occurs in the stem cell populations that produce mature lung cells, ensuring that the effect is stable.
Getting specific
The foundation of the new work is the technology that gets the mRNAs of the COVID-19 mRNA vaccines inside cells. The nucleic acids of an mRNA are large molecules with a lot of charged pieces, which makes it difficult for them to cross a membrane to get inside of a cell. To overcome that problem, the researchers package the mRNA inside a bubble of lipids, which can then fuse with cell membranes, dumping the mRNA inside the cell.
This process, as the researchers note, has two very large advantages: We know it works, and we know it’s safe. “More than a billion doses of lipid nanoparticle–mRNA COVID-19 vaccines have been administered intramuscularly worldwide,” they write, “demonstrating high safety and efficacy sustained through repeatable dosing.” (As an aside, it’s interesting to contrast the research community’s view of the mRNA vaccines to the conspiracies that circulate widely among the public.)
There’s one big factor that doesn’t matter for vaccine delivery but does matter for gene editing: They’re not especially fussy about what cells they target for delivery. So, if you want to target something like blood stem cells, then you need to alter the lipid particles in some way to get them to preferentially target the cells of your choice.
There are a lot of ideas on how to do this, but the team behind this new work found a relatively simple one: changing the amount of positively charged lipids on the particle. In 2020, they published a paper in which they describe the development of selective organ targeting (SORT) lipid nanoparticles. By default, many of the lipid particles end up in the liver. But, as the fraction of positively charged lipids increases, the targeting shifts to the spleen and then to the lung.
So, presumably, because they know they can target the lung, they decided to use SORT particles to send a gene editing system specific to cystic fibrosis, which primarily affects that tissue and is caused by mutations in a single gene. While it’s relatively easy to get things into the lung, it’s tough to get them to lung cells, given all the mucus, cilia, and immune cells that are meant to take care of foreign items in the lung.
IV infusion enables editing of the cystic fibrosis gene in lung stem cells Read More »