FLUX: This new AI image generator is eerily good at creating human hands
five-finger salute —
FLUX.1 is the open-weights heir apparent to Stable Diffusion, turning text into images.
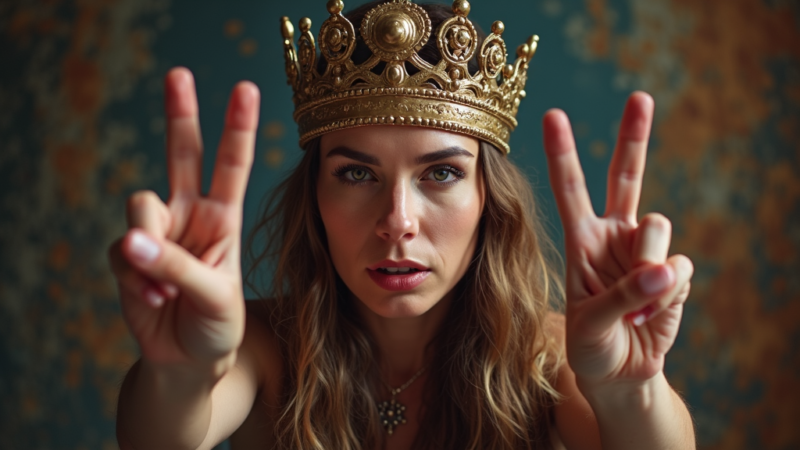
Enlarge / AI-generated image by FLUX.1 dev: “A beautiful queen of the universe holding up her hands, face in the background.”
FLUX.1
On Thursday, AI-startup Black Forest Labs announced the launch of its company and the release of its first suite of text-to-image AI models, called FLUX.1. The German-based company, founded by researchers who developed the technology behind Stable Diffusion and invented the latent diffusion technique, aims to create advanced generative AI for images and videos.
The launch of FLUX.1 comes about seven weeks after Stability AI’s troubled release of Stable Diffusion 3 Medium in mid-June. Stability AI’s offering faced widespread criticism among image-synthesis hobbyists for its poor performance in generating human anatomy, with users sharing examples of distorted limbs and bodies across social media. That problematic launch followed the earlier departure of three key engineers from Stability AI—Robin Rombach, Andreas Blattmann, and Dominik Lorenz—who went on to found Black Forest Labs along with latent diffusion co-developer Patrick Esser and others.
Black Forest Labs launched with the release of three FLUX.1 text-to-image models: a high-end commercial “pro” version, a mid-range “dev” version with open weights for non-commercial use, and a faster open-weights “schnell” version (“schnell” means quick or fast in German). Black Forest Labs claims its models outperform existing options like Midjourney and DALL-E in areas such as image quality and adherence to text prompts.
-
AI-generated image by FLUX.1 dev: “A close-up photo of a pair of hands holding a plate full of pickles.”
FLUX.1
-
AI-generated image by FLUX.1 dev: A hand holding up five fingers with a starry background.
FLUX.1
-
AI-generated image by FLUX.1 dev: “An Ars Technica reader sitting in front of a computer monitor. The screen shows the Ars Technica website.”
FLUX.1
-
AI-generated image by FLUX.1 dev: “a boxer posing with fists raised, no gloves.”
FLUX.1
-
AI-generated image by FLUX.1 dev: “An advertisement for ‘Frosted Prick’ cereal.”
FLUX.1
-
AI-generated image of a happy woman in a bakery baking a cake by FLUX.1 dev.
FLUX.1
-
AI-generated image by FLUX.1 dev: “An advertisement for ‘Marshmallow Menace’ cereal.”
FLUX.1
-
AI-generated image of “A handsome Asian influencer on top of the Empire State Building, instagram” by FLUX.1 dev.
FLUX.1
In our experience, the outputs of the two higher-end FLUX.1 models are generally comparable with OpenAI’s DALL-E 3 in prompt fidelity, with photorealism that seems close to Midjourney 6. They represent a significant improvement over Stable Diffusion XL, the team’s last major release under Stability (if you don’t count SDXL Turbo).
The FLUX.1 models use what the company calls a “hybrid architecture” combining transformer and diffusion techniques, scaled up to 12 billion parameters. Black Forest Labs said it improves on previous diffusion models by incorporating flow matching and other optimizations.
FLUX.1 seems competent at generating human hands, which was a weak spot in earlier image-synthesis models like Stable Diffusion 1.5 due to a lack of training images that focused on hands. Since those early days, other AI image generators like Midjourney have mastered hands as well, but it’s notable to see an open-weights model that renders hands relatively accurately in various poses.
We downloaded the weights file to the FLUX.1 dev model from GitHub, but at 23GB, it won’t fit in the 12GB VRAM of our RTX 3060 card, so it will need quantization to run locally (reducing its size), which reportedly (through chatter on Reddit) some people have already had success with.
Instead, we experimented with FLUX.1 models on AI cloud-hosting platforms Fal and Replicate, which cost money to use, though Fal offers some free credits to start.
Black Forest looks ahead
Black Forest Labs may be a new company, but it’s already attracting funding from investors. It recently closed a $31 million Series Seed funding round led by Andreessen Horowitz, with additional investments from General Catalyst and MätchVC. The company also brought on high-profile advisers, including entertainment executive and former Disney President Michael Ovitz and AI researcher Matthias Bethge.
“We believe that generative AI will be a fundamental building block of all future technologies,” the company stated in its announcement. “By making our models available to a wide audience, we want to bring its benefits to everyone, educate the public and enhance trust in the safety of these models.”
-
AI-generated image by FLUX.1 dev: A cat in a car holding a can of beer that reads, ‘AI Slop.’
FLUX.1
-
AI-generated image by FLUX.1 dev: Mickey Mouse and Spider-Man singing to each other.
FLUX.1
-
AI-generated image by FLUX.1 dev: “a muscular barbarian with weapons beside a CRT television set, cinematic, 8K, studio lighting.”
FLUX.1
-
AI-generated image of a flaming cheeseburger created by FLUX.1 dev.
FLUX.1
-
AI-generated image by FLUX.1 dev: “Will Smith eating spaghetti.”
FLUX.1
-
AI-generated image by FLUX.1 dev: “a muscular barbarian with weapons beside a CRT television set, cinematic, 8K, studio lighting. The screen reads ‘Ars Technica.'”
FLUX.1
-
AI-generated image by FLUX.1 dev: “An advertisement for ‘Burt’s Grenades’ cereal.”
FLUX.1
-
AI-generated image by FLUX.1 dev: “A close-up photo of a pair of hands holding a plate that contains a portrait of the queen of the universe”
FLUX.1
Speaking of “trust and safety,” the company did not mention where it obtained the training data that taught the FLUX.1 models how to generate images. Judging by the outputs we could produce with the model that included depictions of copyrighted characters, Black Forest Labs likely used a huge unauthorized image scrape of the Internet, possibly collected by LAION, an organization that collected the datasets that trained Stable Diffusion. This is speculation at this point. While the underlying technological achievement of FLUX.1 is notable, it feels likely that the team is playing fast and loose with the ethics of “fair use” image scraping much like Stability AI did. That practice may eventually attract lawsuits like those filed against Stability AI.
Though text-to-image generation is Black Forest’s current focus, the company plans to expand into video generation next, saying that FLUX.1 will serve as the foundation of a new text-to-video model in development, which will compete with OpenAI’s Sora, Runway’s Gen-3 Alpha, and Kuaishou’s Kling in a contest to warp media reality on demand. “Our video models will unlock precise creation and editing at high definition and unprecedented speed,” the Black Forest announcement claims.
FLUX: This new AI image generator is eerily good at creating human hands Read More »