Nonprofit scrubs illegal content from controversial AI training dataset
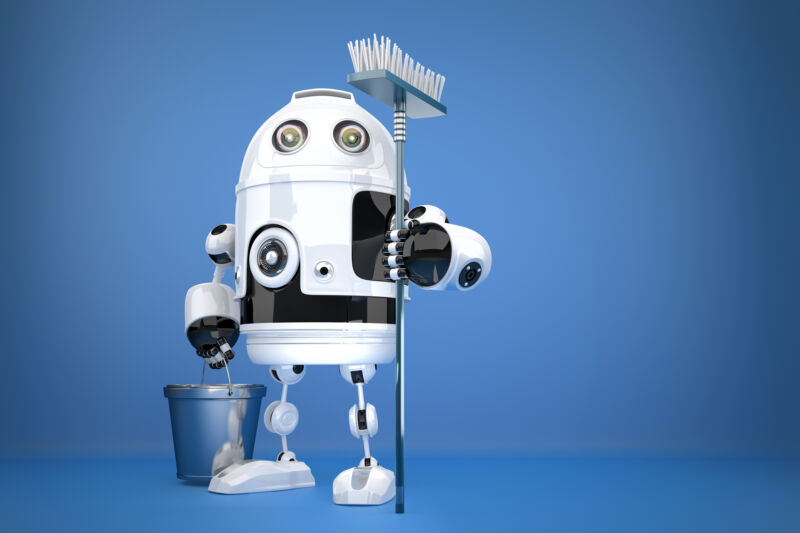
After Stanford Internet Observatory researcher David Thiel found links to child sexual abuse materials (CSAM) in an AI training dataset tainting image generators, the controversial dataset was immediately taken down in 2023.
Now, the LAION (Large-scale Artificial Intelligence Open Network) team has released a scrubbed version of the LAION-5B dataset called Re-LAION-5B and claimed that it “is the first web-scale, text-link to images pair dataset to be thoroughly cleaned of known links to suspected CSAM.”
To scrub the dataset, LAION partnered with the Internet Watch Foundation (IWF) and the Canadian Center for Child Protection (C3P) to remove 2,236 links that matched with hashed images in the online safety organizations’ databases. Removals include all the links flagged by Thiel, as well as content flagged by LAION’s partners and other watchdogs, like Human Rights Watch, which warned of privacy issues after finding photos of real kids included in the dataset without their consent.
In his study, Thiel warned that “the inclusion of child abuse material in AI model training data teaches tools to associate children in illicit sexual activity and uses known child abuse images to generate new, potentially realistic child abuse content.”
Thiel urged LAION and other researchers scraping the Internet for AI training data that a new safety standard was needed to better filter out not just CSAM, but any explicit imagery that could be combined with photos of children to generate CSAM. (Recently, the US Department of Justice pointedly said that “CSAM generated by AI is still CSAM.”)
While LAION’s new dataset won’t alter models that were trained on the prior dataset, LAION claimed that Re-LAION-5B sets “a new safety standard for cleaning web-scale image-link datasets.” Where before illegal content “slipped through” LAION’s filters, the researchers have now developed an improved new system “for identifying and removing illegal content,” LAION’s blog said.
Thiel told Ars that he would agree that LAION has set a new safety standard with its latest release, but “there are absolutely ways to improve it.” However, “those methods would require possession of all original images or a brand new crawl,” and LAION’s post made clear that it only utilized image hashes and did not conduct a new crawl that could have risked pulling in more illegal or sensitive content. (On Threads, Thiel shared more in-depth impressions of LAION’s effort to clean the dataset.)
LAION warned that “current state-of-the-art filters alone are not reliable enough to guarantee protection from CSAM in web scale data composition scenarios.”
“To ensure better filtering, lists of hashes of suspected links or images created by expert organizations (in our case, IWF and C3P) are suitable choices,” LAION’s blog said. “We recommend research labs and any other organizations composing datasets from the public web to partner with organizations like IWF and C3P to obtain such hash lists and use those for filtering. In the longer term, a larger common initiative can be created that makes such hash lists available for the research community working on dataset composition from web.”
According to LAION, the bigger concern is that some links to known CSAM scraped into a 2022 dataset are still active more than a year later.
“It is a clear hint that law enforcement bodies have to intensify the efforts to take down domains that host such image content on public web following information and recommendations by organizations like IWF and C3P, making it a safer place, also for various kinds of research related activities,” LAION’s blog said.
HRW researcher Hye Jung Han praised LAION for removing sensitive data that she flagged, while also urging more interventions.
“LAION’s responsive removal of some children’s personal photos from their dataset is very welcome, and will help to protect these children from their likenesses being misused by AI systems,” Han told Ars. “It’s now up to governments to pass child data protection laws that would protect all children’s privacy online.”
Although LAION’s blog said that the content removals represented an “upper bound” of CSAM that existed in the initial dataset, AI specialist and Creative.AI co-founder Alex Champandard told Ars that he’s skeptical that all CSAM was removed.
“They only filter out previously identified CSAM, which is only a partial solution,” Champandard told Ars. “Statistically speaking, most instances of CSAM have likely never been reported nor investigated by C3P or IWF. A more reasonable estimate of the problem is about 25,000 instances of things you’d never want to train generative models on—maybe even 50,000.”
Champandard agreed with Han that more regulations are needed to protect people from AI harms when training data is scraped from the web.
“There’s room for improvement on all fronts: privacy, copyright, illegal content, etc.,” Champandard said. Because “there are too many data rights being broken with such web-scraped datasets,” Champandard suggested that datasets like LAION’s won’t “stand the test of time.”
“LAION is simply operating in the regulatory gap and lag in the judiciary system until policymakers realize the magnitude of the problem,” Champandard said.
Nonprofit scrubs illegal content from controversial AI training dataset Read More »