OpenAI drops login requirements for ChatGPT’s free version
free as in beer? —
ChatGPT 3.5 still falls far short of GPT-4, and other models surpassed it long ago.
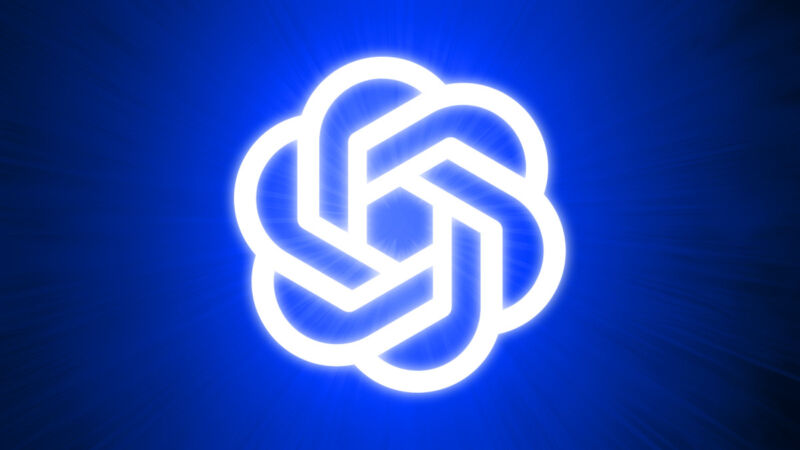
Benj Edwards
On Monday, OpenAI announced that visitors to the ChatGPT website in some regions can now use the AI assistant without signing in. Previously, the company required that users create an account to use it, even with the free version of ChatGPT that is currently powered by the GPT-3.5 AI language model. But as we have noted in the past, GPT-3.5 is widely known to provide more inaccurate information compared to GPT-4 Turbo, available in paid versions of ChatGPT.
Since its launch in November 2022, ChatGPT has transformed over time from a tech demo to a comprehensive AI assistant, and it’s always had a free version available. The cost is free because “you’re the product,” as the old saying goes. Using ChatGPT helps OpenAI gather data that will help the company train future AI models, although free users and ChatGPT Plus subscription members can both opt out of allowing the data they input into ChatGPT to be used for AI training. (OpenAI says it never trains on inputs from ChatGPT Team and Enterprise members at all).
Opening ChatGPT to everyone could provide a frictionless on-ramp for people who might use it as a substitute for Google Search or potentially gain new customers by providing an easy way for people to use ChatGPT quickly, then offering an upsell to paid versions of the service.
“It’s core to our mission to make tools like ChatGPT broadly available so that people can experience the benefits of AI,” OpenAI says on its blog page. “For anyone that has been curious about AI’s potential but didn’t want to go through the steps to set up an account, start using ChatGPT today.”
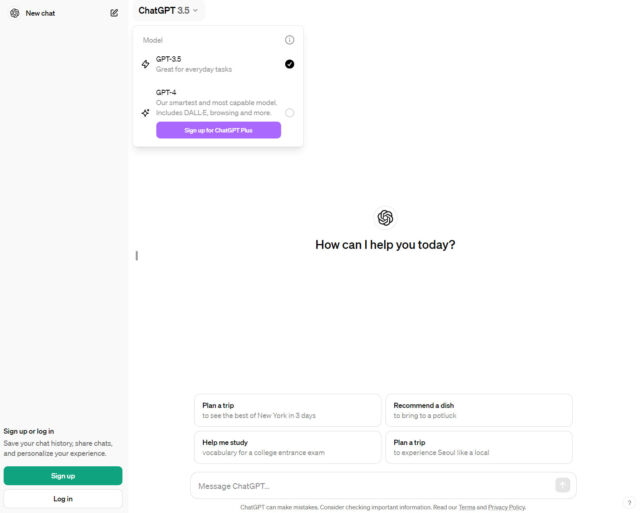
Enlarge / When you visit the ChatGPT website, you’re immediately presented with a chat box like this (in some regions). Screenshot captured April 1, 2024.
Benj Edwards
Since kids will also be able to use ChatGPT without an account—despite it being against the terms of service—OpenAI also says it’s introducing “additional content safeguards,” such as blocking more prompts and “generations in a wider range of categories.” What exactly that entails has not been elaborated upon by OpenAI, but we reached out to the company for comment.
There might be a few other downsides to the fully open approach. On X, AI researcher Simon Willison wrote about the potential for automated abuse as a way to get around paying for OpenAI’s services: “I wonder how their scraping prevention works? I imagine the temptation for people to abuse this as a free 3.5 API will be pretty strong.”
With fierce competition, more GPT-3.5 access may backfire
Willison also mentioned a common criticism of OpenAI (as voiced in this case by Wharton professor Ethan Mollick) that people’s ideas about what AI models can do have so far largely been influenced by GPT-3.5, which, as we mentioned, is far less capable and far more prone to making things up than the paid version of ChatGPT that uses GPT-4 Turbo.
“In every group I speak to, from business executives to scientists, including a group of very accomplished people in Silicon Valley last night, much less than 20% of the crowd has even tried a GPT-4 class model,” wrote Mollick in a tweet from early March.
With models like Google Gemini Pro 1.5 and Anthropic Claude 3 potentially surpassing OpenAI’s best proprietary model at the moment —and open weights AI models eclipsing the free version of ChatGPT—allowing people to use GPT-3.5 might not be putting OpenAI’s best foot forward. Microsoft Copilot, powered by OpenAI models, also supports a frictionless, no-login experience, but it allows access to a model based on GPT-4. But Gemini currently requires a sign-in, and Anthropic sends a login code through email.
For now, OpenAI says the login-free version of ChatGPT is not yet available to everyone, but it will be coming soon: “We’re rolling this out gradually, with the aim to make AI accessible to anyone curious about its capabilities.”
OpenAI drops login requirements for ChatGPT’s free version Read More »