Deepfakes in the courtroom: US judicial panel debates new AI evidence rules
adventures in 21st-century justice —
Panel of eight judges confronts deep-faking AI tech that may undermine legal trials.
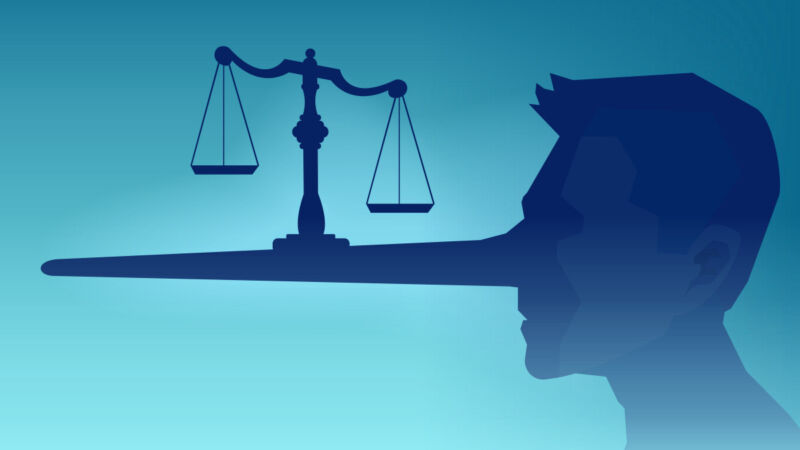
On Friday, a federal judicial panel convened in Washington, DC, to discuss the challenges of policing AI-generated evidence in court trials, according to a Reuters report. The US Judicial Conference’s Advisory Committee on Evidence Rules, an eight-member panel responsible for drafting evidence-related amendments to the Federal Rules of Evidence, heard from computer scientists and academics about the potential risks of AI being used to manipulate images and videos or create deepfakes that could disrupt a trial.
The meeting took place amid broader efforts by federal and state courts nationwide to address the rise of generative AI models (such as those that power OpenAI’s ChatGPT or Stability AI’s Stable Diffusion), which can be trained on large datasets with the aim of producing realistic text, images, audio, or videos.
In the published 358-page agenda for the meeting, the committee offers up this definition of a deepfake and the problems AI-generated media may pose in legal trials:
A deepfake is an inauthentic audiovisual presentation prepared by software programs using artificial intelligence. Of course, photos and videos have always been subject to forgery, but developments in AI make deepfakes much more difficult to detect. Software for creating deepfakes is already freely available online and fairly easy for anyone to use. As the software’s usability and the videos’ apparent genuineness keep improving over time, it will become harder for computer systems, much less lay jurors, to tell real from fake.
During Friday’s three-hour hearing, the panel wrestled with the question of whether existing rules, which predate the rise of generative AI, are sufficient to ensure the reliability and authenticity of evidence presented in court.
Some judges on the panel, such as US Circuit Judge Richard Sullivan and US District Judge Valerie Caproni, reportedly expressed skepticism about the urgency of the issue, noting that there have been few instances so far of judges being asked to exclude AI-generated evidence.
“I’m not sure that this is the crisis that it’s been painted as, and I’m not sure that judges don’t have the tools already to deal with this,” said Judge Sullivan, as quoted by Reuters.
Last year, Chief US Supreme Court Justice John Roberts acknowledged the potential benefits of AI for litigants and judges, while emphasizing the need for the judiciary to consider its proper uses in litigation. US District Judge Patrick Schiltz, the evidence committee’s chair, said that determining how the judiciary can best react to AI is one of Roberts’ priorities.
In Friday’s meeting, the committee considered several deepfake-related rule changes. In the agenda for the meeting, US District Judge Paul Grimm and attorney Maura Grossman proposed modifying Federal Rule 901(b)(9) (see page 5), which involves authenticating or identifying evidence. They also recommended the addition of a new rule, 901(c), which might read:
901(c): Potentially Fabricated or Altered Electronic Evidence. If a party challenging the authenticity of computer-generated or other electronic evidence demonstrates to the court that it is more likely than not either fabricated, or altered in whole or in part, the evidence is admissible only if the proponent demonstrates that its probative value outweighs its prejudicial effect on the party challenging the evidence.
The panel agreed during the meeting that this proposal to address concerns about litigants challenging evidence as deepfakes did not work as written and that it will be reworked before being reconsidered later.
Another proposal by Andrea Roth, a law professor at the University of California, Berkeley, suggested subjecting machine-generated evidence to the same reliability requirements as expert witnesses. However, Judge Schiltz cautioned that such a rule could hamper prosecutions by allowing defense lawyers to challenge any digital evidence without establishing a reason to question it.
For now, no definitive rule changes have been made, and the process continues. But we’re witnessing the first steps of how the US justice system will adapt to an entirely new class of media-generating technology.
Putting aside risks from AI-generated evidence, generative AI has led to embarrassing moments for lawyers in court over the past two years. In May 2023, US lawyer Steven Schwartz of the firm Levidow, Levidow, & Oberman apologized to a judge for using ChatGPT to help write court filings that inaccurately cited six nonexistent cases, leading to serious questions about the reliability of AI in legal research. Also, in November, a lawyer for Michael Cohen cited three fake cases that were potentially influenced by a confabulating AI assistant.
Deepfakes in the courtroom: US judicial panel debates new AI evidence rules Read More »