OpenAI’s new “CriticGPT” model is trained to criticize GPT-4 outputs
automated critic —
Research model catches bugs in AI-generated code, improving human oversight of AI.
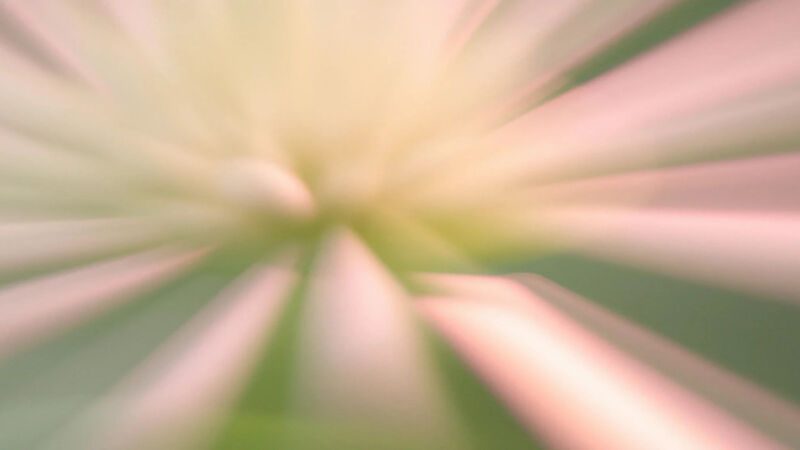
Enlarge / An illustration created by OpenAI.
On Thursday, OpenAI researchers unveiled CriticGPT, a new AI model designed to identify mistakes in code generated by ChatGPT. It aims to enhance the process of making AI systems behave in ways humans want (called “alignment”) through Reinforcement Learning from Human Feedback (RLHF), which helps human reviewers make large language model (LLM) outputs more accurate.
As outlined in a new research paper called “LLM Critics Help Catch LLM Bugs,” OpenAI created CriticGPT to act as an AI assistant to human trainers who review programming code generated by the ChatGPT AI assistant. CriticGPT—based on the GPT-4 family of LLMS—analyzes the code and points out potential errors, making it easier for humans to spot mistakes that might otherwise go unnoticed. The researchers trained CriticGPT on a dataset of code samples with intentionally inserted bugs, teaching it to recognize and flag various coding errors.
The researchers found that CriticGPT’s critiques were preferred by annotators over human critiques in 63 percent of cases involving naturally occurring LLM errors and that human-machine teams using CriticGPT wrote more comprehensive critiques than humans alone while reducing confabulation (hallucination) rates compared to AI-only critiques.
Developing an automated critic
The development of CriticGPT involved training the model on a large number of inputs containing deliberately inserted mistakes. Human trainers were asked to modify code written by ChatGPT, introducing errors and then providing example feedback as if they had discovered these bugs. This process allowed the model to learn how to identify and critique various types of coding errors.
In experiments, CriticGPT demonstrated its ability to catch both inserted bugs and naturally occurring errors in ChatGPT’s output. The new model’s critiques were preferred by trainers over those generated by ChatGPT itself in 63 percent of cases involving natural bugs (the aforementioned statistic). This preference was partly due to CriticGPT producing fewer unhelpful “nitpicks” and generating fewer false positives, or hallucinated problems.
The researchers also created a new technique they call Force Sampling Beam Search (FSBS). This method helps CriticGPT write more detailed reviews of code. It lets the researchers adjust how thorough CriticGPT is in looking for problems, while also controlling how often it might make up issues that don’t really exist. They can tweak this balance depending on what they need for different AI training tasks.
Interestingly, the researchers found that CriticGPT’s capabilities extend beyond just code review. In their experiments, they applied the model to a subset of ChatGPT training data that had previously been rated as flawless by human annotators. Surprisingly, CriticGPT identified errors in 24 percent of these cases—errors that were subsequently confirmed by human reviewers. OpenAI thinks this demonstrates the model’s potential to generalize to non-code tasks and highlights its ability to catch subtle mistakes that even careful human evaluation might miss.
Despite its promising results, like all AI models, CriticGPT has limitations. The model was trained on relatively short ChatGPT answers, which may not fully prepare it for evaluating longer, more complex tasks that future AI systems might tackle. Additionally, while CriticGPT reduces confabulations, it doesn’t eliminate them entirely, and human trainers can still make labeling mistakes based on these false outputs.
The research team acknowledges that CriticGPT is most effective at identifying errors that can be pinpointed in one specific location within the code. However, real-world mistakes in AI outputs can often be spread across multiple parts of an answer, presenting a challenge for future iterations of the model.
OpenAI plans to integrate CriticGPT-like models into its RLHF labeling pipeline, providing its trainers with AI assistance. For OpenAI, it’s a step toward developing better tools for evaluating outputs from LLM systems that may be difficult for humans to rate without additional support. However, the researchers caution that even with tools like CriticGPT, extremely complex tasks or responses may still prove challenging for human evaluators—even those assisted by AI.
OpenAI’s new “CriticGPT” model is trained to criticize GPT-4 outputs Read More »