Evidence of “snowball Earth” found in ancient rocks
On ice —
An outcrop in Scotland has material from when the Earth went into a deep freeze.
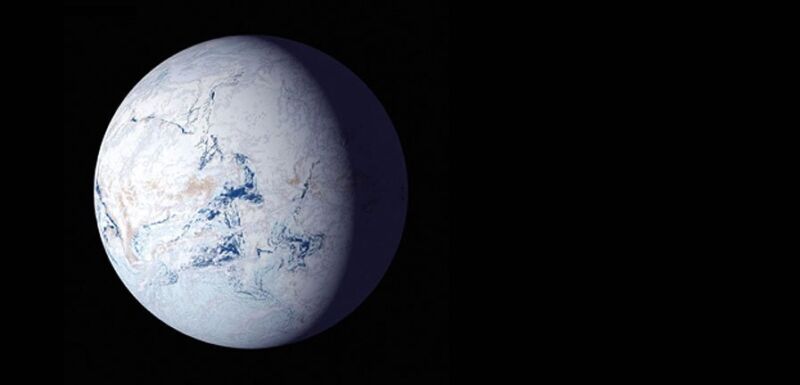
Enlarge / Artist’s conception of the state of the Earth during its global glaciations.
Earth has gone through many geologic phases, but it did have one striking period of stasis: Our planet experienced a tropical environment where algae and single-celled organisms flourished for almost 2 billion years. Then things changed drastically as the planet was plunged into a deep freeze.
It was previously unclear when Earth became a gargantuan freezer. Now, University College London researchers have found evidence in an outcrop of rocks in Scotland, known as the Port Askaig Formation, that show evidence of the transition from a tropical Earth to a frozen one 717 million years ago. This marks the onset of the Sturtian glaciation and would be the first of two “snowball Earth” events during which much of the planet’s surface was covered in ice. It is thought that multicellular life began to emerge after Earth thawed.
Found in the Scottish islands known as the Garvellachs, this outcrop within the Port Askaig Formation is unique because it offers the first conclusive evidence of when a tropical Earth froze over—underlying layers that are a timeline from a warmer era to a frigid one. Other rocks that formed during the same time period in other parts of the world lack this transitional evidence because ancient glaciers most likely scraped it off.
“The Port Askaig preserves a relatively complete record of the global “Sturtian glaciation,” the researchers said in a study recently published in the Journal of the Geological Society.
In my snowball era
Underneath the rocks that formed during the Sturtian glaciation is a deep layer of carbonate rocks known as the Garb Eileach Formation. These were dated to the warm, tropical Tonian period, which started 1 billion years ago and lasted until 717 million years ago, when the cold took over. The youngest rocks in this formation are evidence of the transition to the first “snowball Earth.”
Why did Earth endure such a big chill to begin with? A sudden decrease in solar radiation probably led to an especially long winter that set off a (if you’ll pardon the pun) snowball effect. With less radiation, more ice forms, and more ice makes the planet more reflective, meaning it sends more sunlight back into space and causes the planet to continue cooling, allowing even more ice to form.
To find out when this global chill began, the research team collected 11 sandstone samples from the Garvellach Islands to analyze zircons in the sandstone. Zircons are especially useful in dating rock formations because they are often as old as the rock they are in, making some of them the oldest minerals on Earth. They also resist being chemically degraded. What is especially important about zircons is that they contain uranium, which decays into lead over long periods. The amount of uranium that has changed to lead can tell us the amount of time that has passed since the zircon’s formation.
Just a phase
Using both laser ablation (a type of laser imaging that reveals how elements and isotopes are distributed in a sample) and plasma mass spectrometry, the researchers determined the uranium-lead ratio. The time it had taken for the uranium to become lead was in line with their estimates, which were based on previous studies that had estimated, but not confirmed, the time of onset for the Sturtian glaciation.
Another thing that the outcrop’s zircons told the researchers is that the Sturtian glaciation lasted around 58 million years. It was closely followed by the Marinoan glaciation, thought to have lasted another 16 million years, and both these “snowball Earth” phases make up what is known as the Cryogenic period. The rocks containing these zircons were probably deposited by a moving glacier as the supercontinent Rodinia (which preceded the more famous Pangaea) was breaking apart.
The Port Askaig Formation is now, as the scientists say in the same study, “one of the thickest (up to 1.1 km) and most complete records of Cryogenic glaciation.”
Because glaciers did not scrape this formation away, a record of when Earth started to warm up again is also preserved. The zircon crystals that formed during the Sturtian glaciation gradually disappear in younger rocks until they are replaced by zircons formed after the ice began to melt. So not only is there evidence of the beginning of the deep freeze, but also evidence for the thaw that began around 635 million years ago.
As the ice melted, complex multicellular life began to burst onto the scene during the Ediacaran period. There could be several reasons for this. Hypotheses suggest that the temperature of the seawater rose, an influx of sunlight sparked photosynthesis, and there was a greater availability of nutrients than before.
The scientists involved in the Port Askaig study think that any life that survived the Cryogenian period faced an immense challenge once the ice began to melt. These organisms had been used to perpetual cold for millions of years, and now they faced the struggle of adapting as soon as possible—or perishing.
What about those that survived? They ended up being the ancestors of all animals that ever existed, and that includes us.
Journal of the Geological Society, 2024. DOI: 10.1144/jgs2024-02
Evidence of “snowball Earth” found in ancient rocks Read More »