Texas judge decides Texas is a perfectly good venue for X to sue Media Matters
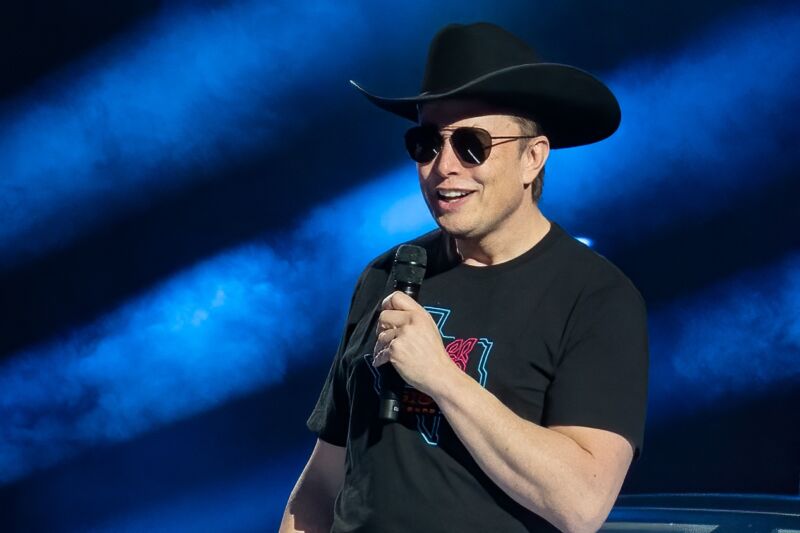
Enlarge / Tesla CEO Elon Musk speaks at Tesla’s “Cyber Rodeo” on April 7, 2022, in Austin, Texas.
Getty Images | AFP/Suzanne Cordeiro
A federal judge in Texas yesterday ruled that Elon Musk’s X Corp. can continue its lawsuit against Media Matters for America. US District Judge Reed O’Connor of the Northern District of Texas, who recently refused to recuse himself from the case despite having purchased Tesla stock, denied Media Matters’ motion to dismiss.
X Corp. sued Media Matters after the nonprofit watchdog group published research on ads being placed next to pro-Nazi content on X, formerly Twitter. X’s lawsuit also names reporter Eric Hananoki and Media Matters President Angelo Carusone as defendants.
Because of O’Connor’s ruling, X can move ahead with its claims of tortious interference with contract, business disparagement, and tortious interference with prospective economic advantage. A jury trial is scheduled to begin on April 7, 2025.
“Plaintiff alleges that Defendants knowingly and maliciously fabricated side-by-side images of various advertisers’ posts on Plaintiff’s social media platform X depicted next to neo-Nazi or other extremist content, and portrayed these designed images as if they were what the average user experiences on the X platform,” O’Connor wrote in his ruling on the motion to dismiss. “Plaintiff asserts that Defendants proceeded with this course of action in an effort to publicly portray X as a social media platform dominated by neo-Nazism and anti-Semitism, and thereby alienate major advertisers, publishers, and users away from the X platform, intending to harm it.”
A different federal judge in the District of Columbia recently criticized X’s claims, pointing out that “X did not deny that advertising in fact had appeared next to the extremist posts on the day in question.” But X has a more friendly judge in O’Connor, who has made several rulings against Media Matters. The defendant could also face a tough road on appeal because challenges would go to the conservative-leaning US Court of Appeals for the 5th Circuit.
Judge: Media Matters “targeted” Texas-based advertisers
Media Matters’ motion to dismiss argues in part that Texas is an improper forum for the dispute because “X is organized under Nevada law and maintains its principal place of business in San Francisco, California, where its own terms of service require users of its platform to litigate any disputes.” (Musk recently said that X will move its headquarters from San Francisco to Austin, Texas.)
O’Connor’s ruling acknowledges that “when a nonresident defendant files a motion to dismiss for lack of personal jurisdiction, the burden of proof is on the plaintiff as the party seeking to invoke the district court’s jurisdiction.” In this case, O’Connor said that jurisdiction is established if the defendants “targeted the conduct that is the basis for this lawsuit at Texas.”
O’Connor ruled that the court has jurisdiction because Media Matters articles “targeted” Texas-based companies that advertised on X, specifically Oracle and AT&T, even though those companies are not parties to the lawsuit. O’Connor said the Media Matters “articles targeted, among others, Oracle, a Texas-based company that placed ads on Plaintiff’s platform… Plaintiff also alleges that this ‘crusade’ targeted its blue-chip advertisers which included Oracle and AT&T, Texas-based companies.”
O’Connor, a George W. Bush appointee, wrote that a “defendant who targets a Texas company with tortious activity has fair warning that it may be sued there.”
“This targeting of the alleged tortious acts at the headquarters of Texas-based companies is sufficient to establish specific jurisdiction in Texas… each Defendant engaged in the alleged tortious acts which targeted harm in, among other places, Texas,” he wrote.
Judge cites TV appearances
That includes Hananoki, the Media Matters reporter who wrote the articles, and Carusone. Each of those individual defendants “targeted” the conduct at Texas, O’Connor found.
“Plaintiff alleges Carusone participated in the ‘crusade’ with Hananoki and Media Matters when he appeared on television shows a number of times discussing the importance of advertisers to Plaintiff’s business model and advocating that advertisers should cease doing business with Plaintiff if there is a deluge of ‘unmoderated right-wing hatred and misinformation,'” O’Connor wrote.
Ruling that “Media Matters targeted Texas,” O’Connor wrote that the group pursued “a strategy to target Plaintiff’s blue-chip advertisers, including Oracle and AT&T, Texas-based companies; in furtherance of this strategy it published the Hananoki articles, and it published other articles pressuring the blue-chip advertisers, all to pressure blue-chip advertisers to cease doing business with Plaintiff. Finally, the inference from Media Matters’ affidavit is that Media Matters also emailed the Hananoki articles to Texans, and Plaintiff’s lawsuit arises out of this conduct.”
Media Matters also sought dismissal on the basis that X failed to state a claim. But O’Connor said that “the Court must accept all well-pleaded facts in the complaint as true and view them in the light most favorable to the plaintiff,” and he found that X “has provided sufficient allegations to survive dismissal.”
Media Matters declined to comment when contacted by Ars today.
Texas judge decides Texas is a perfectly good venue for X to sue Media Matters Read More »