slow and steady —
AlphaProof and AlphaGeometry 2 solve problems, with caveats on time and human assistance.
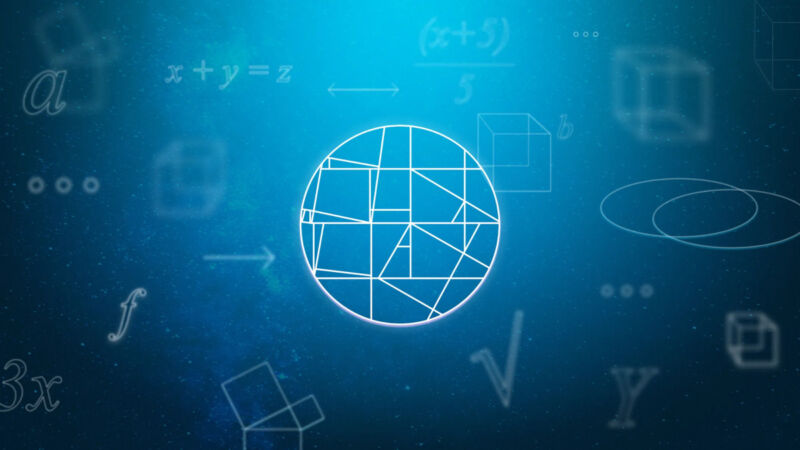
Enlarge / An illustration provided by Google.
On Thursday, Google DeepMind announced that AI systems called AlphaProof and AlphaGeometry 2 reportedly solved four out of six problems from this year’s International Mathematical Olympiad (IMO), achieving a score equivalent to a silver medal. The tech giant claims this marks the first time an AI has reached this level of performance in the prestigious math competition—but as usual in AI, the claims aren’t as clear-cut as they seem.
Google says AlphaProof uses reinforcement learning to prove mathematical statements in the formal language called Lean. The system trains itself by generating and verifying millions of proofs, progressively tackling more difficult problems. Meanwhile, AlphaGeometry 2 is described as an upgraded version of Google’s previous geometry-solving AI modeI, now powered by a Gemini-based language model trained on significantly more data.
According to Google, prominent mathematicians Sir Timothy Gowers and Dr. Joseph Myers scored the AI model’s solutions using official IMO rules. The company reports its combined system earned 28 out of 42 possible points, just shy of the 29-point gold medal threshold. This included a perfect score on the competition’s hardest problem, which Google claims only five human contestants solved this year.
A math contest unlike any other
The IMO, held annually since 1959, pits elite pre-college mathematicians against exceptionally difficult problems in algebra, combinatorics, geometry, and number theory. Performance on IMO problems has become a recognized benchmark for assessing an AI system’s mathematical reasoning capabilities.
Google states that AlphaProof solved two algebra problems and one number theory problem, while AlphaGeometry 2 tackled the geometry question. The AI model reportedly failed to solve the two combinatorics problems. The company claims its systems solved one problem within minutes, while others took up to three days.
Google says it first translated the IMO problems into formal mathematical language for its AI model to process. This step differs from the official competition, where human contestants work directly with the problem statements during two 4.5-hour sessions.
Google reports that before this year’s competition, AlphaGeometry 2 could solve 83 percent of historical IMO geometry problems from the past 25 years, up from its predecessor’s 53 percent success rate. The company claims the new system solved this year’s geometry problem in 19 seconds after receiving the formalized version.
Limitations
Despite Google’s claims, Sir Timothy Gowers offered a more nuanced perspective on the Google DeepMind models in a thread posted on X. While acknowledging the achievement as “well beyond what automatic theorem provers could do before,” Gowers pointed out several key qualifications.
“The main qualification is that the program needed a lot longer than the human competitors—for some of the problems over 60 hours—and of course much faster processing speed than the poor old human brain,” Gowers wrote. “If the human competitors had been allowed that sort of time per problem they would undoubtedly have scored higher.”
Gowers also noted that humans manually translated the problems into the formal language Lean before the AI model began its work. He emphasized that while the AI performed the core mathematical reasoning, this “autoformalization” step was done by humans.
Regarding the broader implications for mathematical research, Gowers expressed uncertainty. “Are we close to the point where mathematicians are redundant? It’s hard to say. I would guess that we’re still a breakthrough or two short of that,” he wrote. He suggested that the system’s long processing times indicate it hasn’t “solved mathematics” but acknowledged that “there is clearly something interesting going on when it operates.”
Even with these limitations, Gowers speculated that such AI systems could become valuable research tools. “So we might be close to having a program that would enable mathematicians to get answers to a wide range of questions, provided those questions weren’t too difficult—the kind of thing one can do in a couple of hours. That would be massively useful as a research tool, even if it wasn’t itself capable of solving open problems.”